Bachelor's Degree Thesis at Cyprus University of Technology.
Access: ktisis.cut.ac.cy
Abstract
Spatial interpolation is the process of calculating unknown values based on some sampling observations and applies to phenomena with constant change in space, therefore is one of the most useful functions used in the science of Geography. This study attempts to develop and examine a methodology to produce Digital Elevation Model (DEM) from a Digital Surface Model (DSM), while investigating the effect of the spatial distribution of the elevation points being used. The methodology proposed aims to automate the DTM production process and minimize the production time, while maintaining a high-quality result and a low-cost approach. The area of study contains the traditional settlement of Vrisa in the island of Lesvos, Greece and the data are gathered after the catastrophic earthquake of June 12th, 2017. Implementation of this study methodology has resulted to the RS_Sampling Tool, a geo-processing tool developed in ArcMap 10, which extracts points with up-to-date altitude information (DSM) according to the parameters set and using the two sampling design proposals (random and stratified sampling). The IDW interpolation method is being used to a workflow with a total of six case studies (differentiating elevation point’s quantity and spatial distribution). Concluding, the following main conclusions appeared: a) The more points being used from the DSM the more accurate is the final DEM, as long as these points are representing pure ground and b) a random sample distribution is much more likely to yield a DEM with low accuracy rather than high. However, a random based distribution can yield a high accuracy DEM, by including high-precision GCP’s.
Project Overview
During the analysis and interpretation of spatial patterns it is important to consider the integral aspect of which is the notion of spatial association, also known as spatial autocorrelation according to Tobler’s first law of geography. According to this law,
“everything is related to everything else, but near things are more related than distant things”
Therefore similar elevation values are expected in neighbouring areas or even similar price value per square meter in neighbouring plots.
This study aims to develop and test a semi-automated method for selecting points from a DSM in order to produce a DEM. Firstly, a UAV flew over the area of interest and the DSM is produced. Using a variety of sampling methods on the DSM, many DTM’s are produced and compared. Next, the effect of the spatial distribution of the elevation points is being investigated by varying the quantity and the randomness of the elevation points used in the spatial interpolation process. In the following chapters the proposed methodology is analysed.
Proposed Methodology
The semi-automated methodology proposed contains the following steps:
DSM production: a DSM of the Area of interest is produced with UAV,
Object-based Classification: all polygons of pure ground on the DSM are isolated,
RS_Sampling geoprocessing Tool: selecting elevation points within the polygons according to the parameters defined (random or stratified sampling, number of points, distance, etc),
Spatial interpolation: DTM production.

Due to the very recent advances in the fields of computer vision and photogrammetry in combination with the improvements in data processing power, orthophoto maps and Digital Surface Models (DSM) can be easily produced, by terrestrial and/or aerial high-resolution 2D imagery.
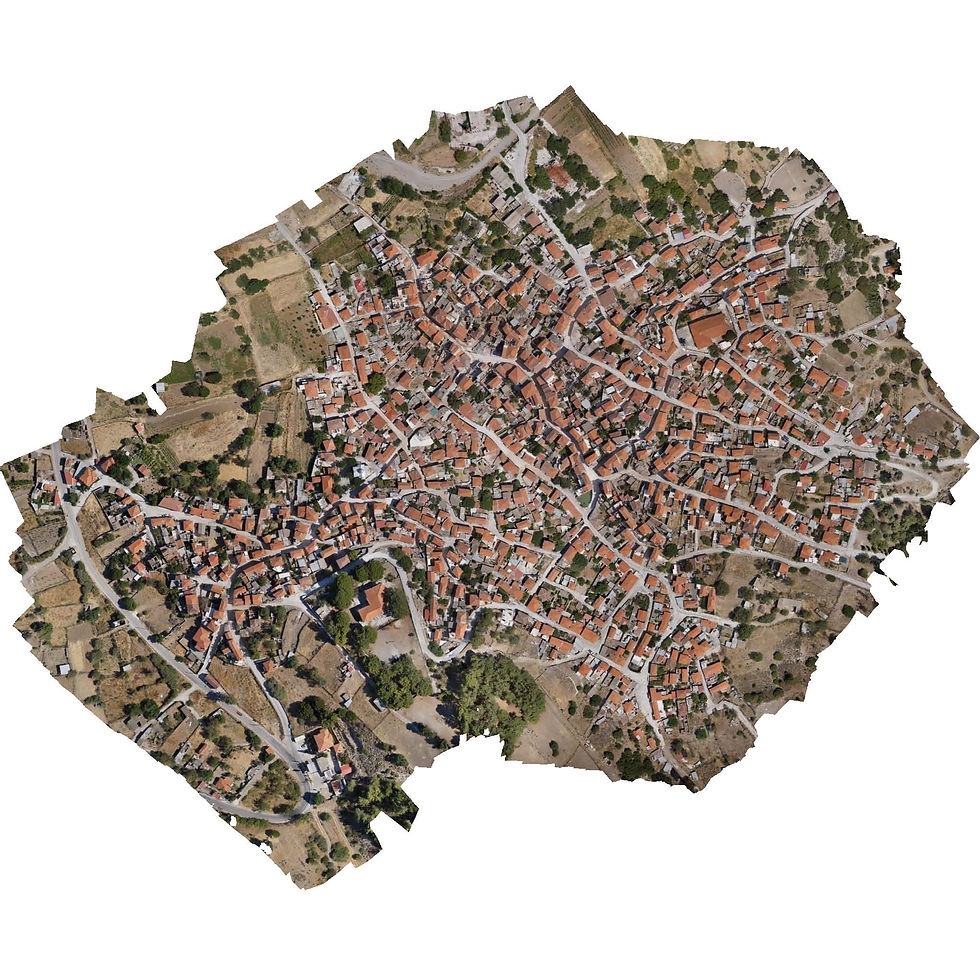

It is necessary to separate the ground areas from which the elevation points will be extracted and used. This is possible using classification methods and two are the most important, a) the pixel-based method, in which classification is done according to the ground spectrum differences and b) the object-based method, in which classification is done relying not only on the spectral characteristics of ground, but also makes further use of geometric and structural information. Many studies, has proven the efficiency and the advantages of the Object-based classification method over the traditional pixel-based method especially in reducing point noises and “salt and pepper” phenomena.

To define only the pure ground areas of the DSM as polygons, the Object-based classification approach is implemented, using the Feature Extraction tool in ENVI 5.0. This led to 1250 polygons of various sizes where 626 are polygons with area less than 10 m2 (named small areas), containing a total of more than 25 million elevation points. After the classification process, polygons have been resulted containing a large number of elevation points.
RS_Sampling Tool
In order to select the appropriate elevation points needed and reduce the total number in order to be used in the spatial interpolation process, a Sampling Design proposal is needed. The RS_Sampling Tool (fig.5) developed implements the Sampling design proposals and exports - according to the parameters set - all the resulting elevation points. The last step of the process is the spatial interpolation.

Results
Applying the IDW interpolation method the following results appeared (fig.6). Comparing the six elevation surfaces, scenario Z has resulted to a much rougher surface and that is due to the fact that all 25 million elevation points are being used.

Conclusions
In this research, a semi-automated methodology for creating a DEM from a DSM is proposed and tested on a real AOI. Furthermore, by applying a total of six point sampling scenarios and with the use of 148 high precision (RTK) GCP’s it was possible to examine the effect of point sampling quantity and distribution on the resulted quality of the products. By comparing the number of elevation points used in each case with the RMSE that resulted (fig.6), it is interesting to observe that as the quantity of points increases the greater is the resulted accuracy (low RMSE).
Comments